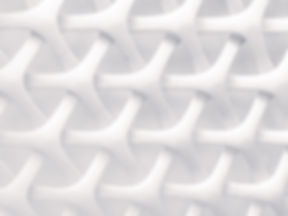
Four years ago, The Economist grabbed our collective attention with the headline: ‘The worlds’ most valuable resource is no longer oil, but data’.
​
Accompanied by an image of oil rigs bearing the logos of Facebook, Google, Amazon, …, we all intuitively nodded our heads.
Sure. Big data was big business for big business.
And then, we went back to our day jobs!
But the reality is that data is everyone’s business, big and small. None more so than in the private equity sector.
Yet, on the face of it, GPs have been slow to harness the transformative power – and value – of data and advanced analytics. However, momentum is building, driven by heightened competition for capital, deals, regulation, and reporting requirements. Plus, of course, the quest for alpha.
​
What are the benefits of advance analytics and data science for GPs?
Clearly, there are many approaches and tools which can improve the operations of GP themselves: from origination to due diligence to investor reporting.
For example, EQT Ventures (the venture arm of Swedish parent EQT Partners) have built a proprietary machine learning platform to support their origination. Aptly named ‘Motherbrain’, it pulls the structured and unstructured data of over 10 million companies, from multiple sources, into a centralised system – enabling deal and operating teams to identify patterns and themes useful to target investments. Motherbrain has been pivotal in the sourcing of several successful investments, including Peakon, which EQT Ventures sold to Workday for US$700m earlier this year.
Likewise, many GPs are using advanced analytics within their due diligence, to assess a target company’s organisational capabilities, customer behaviour, product portfolios, and brand awareness as they seek pre-deal valuation validation.
Furthermore, as more companies and Information Memorandum claim: ‘proprietary AI-backed decision making’ and ‘advanced automation’ – savvy investors are also diving more deeply into the data ecosystems, datasets, and algorithms themselves to determine reality – and then pricing and value creation strategies.
During these deep-dive data due diligences, prospective investors are asking the killer question: How effective is the target company at leveraging data and analytics to power its business and operational models?
​
Portfolio company optimisation & value creation through data
Data – be it a client list, production line feed, vehicle tracking application, ERP, inventory, or workforce management system – can frequently be leveraged beyond its current use, thanks to data-science techniques. For many private equity-owned businesses – which have been heavily optimised using traditional operating methods – leveraging this data might very well be their ‘last mile’ in efficiency.
And I use the word ‘leveraging’ deliberately. Because opex or capex or both have typically been spent implementing the incumbent system and collecting the data. So, the leverage here is that you are using the same data again, just for a different purpose[1]; there’s a compounding effect.
In fact, this is one of the extraordinary characteristics of data (as opposed to other assets) – it can be used again and again, without deteriorating or depreciating. In fact, its value multiplies with each new use and use case.
Of course, it’s easy to assume that these mystical datasets and alchemic data-science techniques are the exclusive domain of sexy start-ups or the deep-pocketed giants on our Economist cover. But the benefits hold true for traditional, analogue business, too. Arguably, the multiplier potential is greater and value extraction opportunity higher!
It’s revelations like these which normally persuade management teams to cross the analytics chasm: shifting from traditional, business intelligence outputs (such as rear-view-mirror reporting) to predictive, data-led insights that can optimise operations, enhance top-line performance, and sharpen competitive edges.
​
Start with the business problem, not the data
Nonetheless, delivering data’s value is hard. Gartner research tells us that 80% of data projects are failing.
One of the chief tactics to avoid becoming this statistic is to tightly link data-science projects to the portfolio company’s business strategy and KPIs, via a data strategy. At a high level, this strategy must frame:
-
The Busines problem – for example: a SaaS business’ problem might be to increase ARR
-
The Analytical solution which solves it – in our SaaS example, this might be a combination of churn, cross-sell and upsell modelling
-
The Data sources and resources required – which might be (i) SalesForce and online customer behaviour data sources, and (ii) domain experts from the portfolio company sales and marketing teams, plus data scientists to build the analytical models
-
A well-defined roadmap – comprising the infrastructure, investment required, quick wins, decision making forums, and success measures.
​​
One of the most sophisticated data-science-led GPs, UK-based HG Capital, has published a case study which powerfully demonstrates the outcomes gained by SaaS portfolio company Access, following the roll out of just such a cross-selling algorithm. After only four months, the project delivered a 25% increase in sales pipeline creation and 30% lift in sales conversion – resulting in a 60% uplift in converted sales orders.
Of course, results like this are never guaranteed. But you’ll be considerably closer to achieving them if you link your portfolio company data projects to business outcomes.
This is the true unlock of data.
​
Building data & advanced analytics capabilities
It’s true, HG Capital have over 30 in-house data scientists embedded into their investment team! I concede that most GPs don’t have the capacity to build such internal capability. Rather, many firms are testing the analytical waters by using specialist data science consultancies to build out their proof-of-concept projects and manage the medium-term outputs, whilst transferring skills.
However, GPs do need to build the skill base of their teams to embrace and embed these new techniques.
After all, consider the new data opportunities arising from (radically) accelerated digital transformation at portfolio companies, following the COVID pandemic. As business models were disrupted and digitally recast, IT systems and customer platforms morphed – almost overnight.
And what do all these digitally evolved systems and processes have in common?
They facilitate the collection and curation of more and more data. Meaning, as the volume, velocity, variety, and veracity of company data increases, so does its potential value.
So, assess it. And realise it.
​
Wilkinson is Co-founder | DataDiligence
​
-
(You’re not having to pay for it twice – though you may require new investment into a data infrastructure and skills).